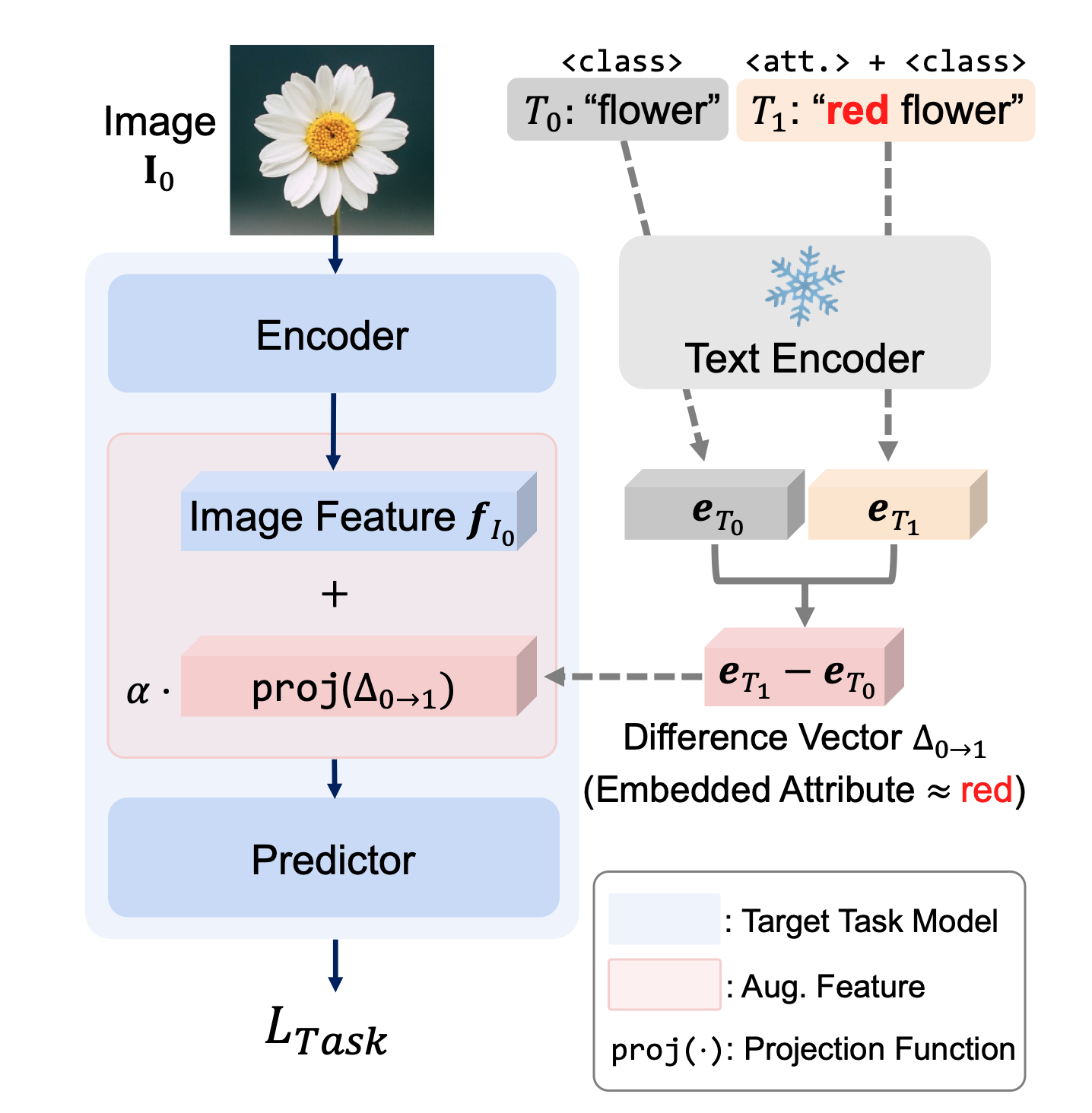
Recent label mix-based augmentation methods have shown their effectiveness in generalization despite their simplicity, and their favorable effects are often attributed to semantic-level augmentation. However, we found that they are vulnerable to highly skewed class distribution, because scarce data classes are rarely sampled for inter-class perturbation.
We propose TextManiA, a text-driven manifold augmentation method that semantically enriches visual feature spaces, regardless of data distribution. TextManiA augments visual data with intra-class semantic perturbation by exploiting easy-to-understand visually mimetic words, i.e., attributes. To this end, we bridge between the text representation and a target visual feature space, and propose an efficient vector augmentation.
To empirically support the validity of our design, we devise two visualization-based analyses and show the plausibility of the bridge between two different modality spaces. Our experiments demonstrate that TextManiA is powerful in scarce samples with class imbalance as well as even distribution. We also show compatibility with the label mix-based approaches in evenly distributed scarce data.
1. Given image $\mathbf{I}_0$ & label $T_0$
2. Synthesize text variant $T_1$
3. Compute difference vector $\mathbf{\Delta}_{0\to1}$
4. Add projected $\mathbf{\Delta}_{0\to1}$ with image feature $\mathbf{f}_{I_0}$
5. Train the model with augmented feature
     $\hat{\mathbf{f}}_{I_0} = \mathbf{f}_{I_0} + \alpha\cdot \mathtt{proj}(\mathbf{\Delta}_{0\to1})$
This work was partly supported by Institute of Information & communications Technology Planning & Evaluation (IITP) grant funded by the Korea government(MSIT) (No.2021-0-02068, Artificial Intelligence Innovation Hub; No.2022-0-00124, Development of Artificial Intelligence Technology for Self-Improving Competency-Aware Learning Capabilities; No. 2020-0-00004, Development of Previsional Intelligence based on Long-term Visual Memory Network)
@inproceedings{yebin2023textmania,
title = {TextManiA: Enriching Visual Feature by Text-driven Manifold Augmentation},
author = {Moon Ye-Bin and Jisoo Kim and Hongyeob Kim and Kilho Son and Tae-Hyun Oh},
booktitle = {ICCV},
year = {2023},
}